CDH 6 includes Apache Kafka as part of the core package. The documentation includes improved contents for how to set up, install, and administer your Kafka ecosystem. For more information, see the Cloudera Enterprise 6.0.x Apache Kafka Guide. We look forward to your feedback on both the existing and new documentation.
CDK Powered By Apache Kafka®
CDK Powered By Apache Kafka® is a distributed commit log service. Kafka functions much like a publish/subscribe messaging system, but with better throughput, built-in partitioning, replication, and fault tolerance. Kafka is a good solution for large scale message processing applications. It is often used in tandem with Apache Hadoop, Apache Storm, and Spark Streaming.
You might think of a log as a time-sorted file or data table. Newer entries are appended to the log over time, from left to right. The log entry number is a convenient replacement for a timestamp.
Kafka integrates this unique abstraction with traditional publish/subscribe messaging concepts (such as producers, consumers, and brokers), parallelism, and enterprise features for improved performance and fault tolerance.
The original use case for Kafka was to track user behavior on websites. Site activity (page views, searches, or other actions users might take) is published to central topics, with one topic per activity type.
Kafka can be used to monitor operational data, aggregating statistics from distributed applications to produce centralized data feeds. It also works well for log aggregation, with low latency and convenient support for multiple data sources.
Kafka provides the following:
- Persistent messaging with O(1) disk structures, meaning that the execution time of Kafka's algorithms is independent of the size of the input. Execution time is constant, even with terabytes of stored messages.
- High throughput, supporting hundreds of thousands of messages per second, even with modest hardware.
- Explicit support for partitioning messages over Kafka servers. It distributes consumption over a cluster of consumer machines while maintaining the order of the message stream.
- Support for parallel data load into Hadoop.
Understanding Kafka Terminology
Kafka and Cloudera Manager use terms in ways that might vary from other technologies. This topic provides definitions for how these terms are used in Kafka with Cloudera Manager.
A service is an application that runs in a CDH cluster. Kafka is a service. ZooKeeper is a service that runs within a Kafka cluster. Other services include MapReduce, HDFS, YARN, Flume, and Spark.
A role is a feature of a service. A broker is a role in a Kafka service.
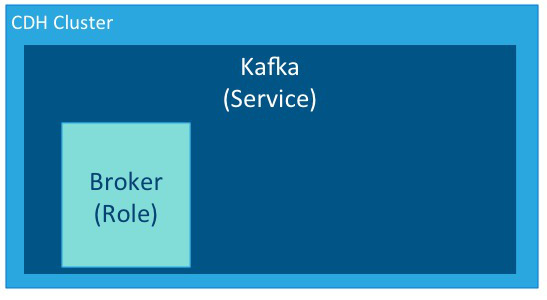
An instance is a deployed and configured software component. A cluster can include multiple roles and multiple instances of the same role. A service instance might be Kafka-1. Kafka-1 might host the role instances Broker-1, Broker-2, and Broker-3.
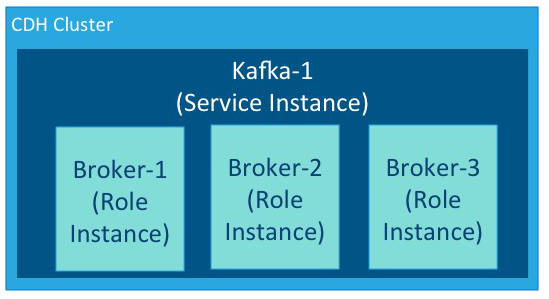
Kafka brokers process records organized into topics. A topic is a category of records that share similar characteristics. For example, a topic might consist of instant messages from social media or navigation information for users on a web site. Each topic has a unique corresponding table in data storage.
A producer is an external process that sends records to a Kafka topic. A consumer is an external process that receives topic streams from a Kafka cluster.
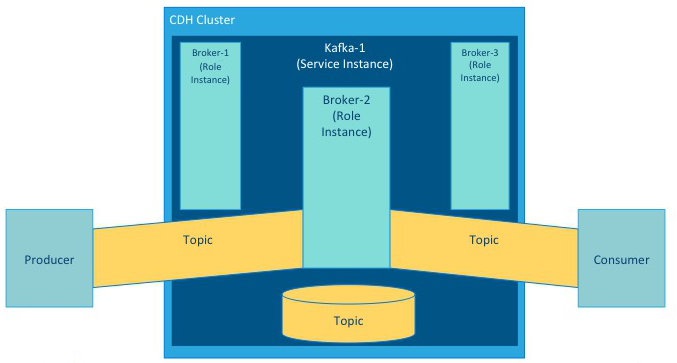
Brokers process topics in partitions. A partition on one broker in a cluster is the leader. The same partition is mirrored on one or more other brokers in the cluster as replicas. When a leader goes offline, a replica automatically takes its place and becomes the new leader for the topic. An in-sync replica is a replica that is completely up-to-date with the leader.
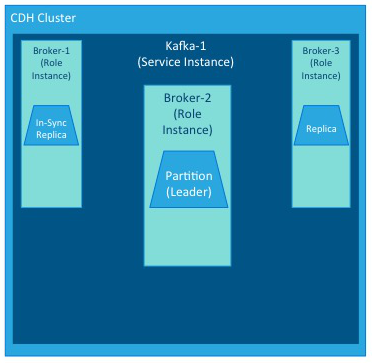
Each Kafka cluster has one broker that also acts as the controller. The controller is responsible for managing the states of partitions and replicas. It also performs administrative tasks, such as reassigning partitions.
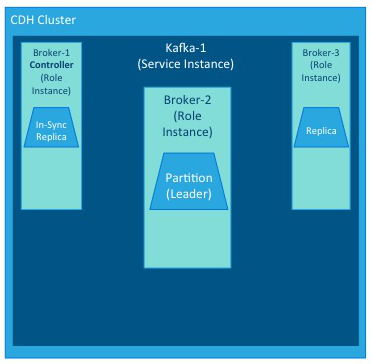
While these illustrations show single instances of the components of a Kafka implementation, Kafka brokers typically host multiple partitions and replicas, with any number of producers and consumers, up to the requirements and limits of the deployed system.
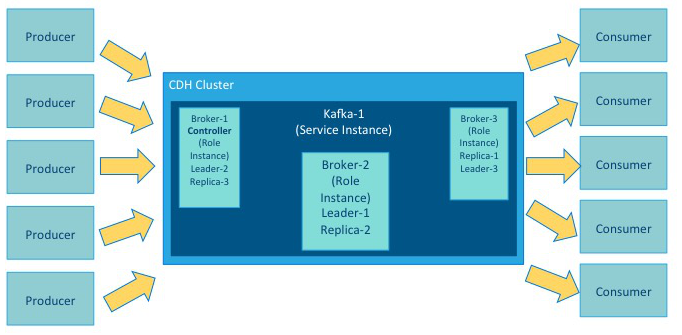