Known Issues and Limitations
There are some known issues you might run into while using Cloudera Machine Learning (CML).
Model build failures when using a custom AWS ECR credential (DSE-35732)
When users follow the guide on the Add Docker registry credentials and certificates page and add their AWS ECS docker credentials to the regcred secret on a CML workspace, model builds fail on that workspace.
- Change the docker image used by the deployment to
container.repository.cloudera.com/cloudera/cdsw/s2i-server:2.0.41-b238
- Change the value of the
BUILDER_IMAGE
environmental variable tocontainer.repository.cloudera.com/cloudera/cdsw/s2i-builder-buildkit:2.0.41-b238
Update button not visible in
tab. (DSE-23572)- API keys expiration in days
- User Web Browser Timeout (minutes)
- Admin User Web Browser Timeout (minutes)
- Root CA configuration
site_config
table.
Perform the following SQL query: SET enable_change_auth_type = 't';
This will make the UI button visible. Web pod crashes if a project forking takes more than 60 minutes (DSE-35251)
2024-04-23 22:52:36.384 1737 ERROR AppServer.VFS.grpc crossCopy grpc error data = [{"error":"1"},{"code":4,"details":"2","metadata":"3"},"Deadline exceeded",{}]
["Error: 4 DEADLINE_EXCEEDED: Deadline exceeded\n at callErrorFromStatus (/home/cdswint/services/web/node_modules/@grpc/grpc-js/build/src/call.js:31:19)\n at Object.onReceiveStatus (/home/cdswint/services/web/node_modules/@grpc/grpc-js/build/src/client.js:192:76)\n at Object.onReceiveStatus (/home/cdswint/services/web/node_modules/@grpc/grpc-js/build/src/client-interceptors.js:360:141)\n at Object.onReceiveStatus (/home/cdswint/services/web/node_modules/@grpc/grpc-js/build/src/client-interceptors.js:323:181)\n at /home/cdswint/services/web/node_modules/@grpc/grpc-js/build/src/resolving-call.js:94:78\n at process.processTicksAndRejections (node:internal/process/task_queues:77:11)\nfor call at\n at ServiceClientImpl.makeUnaryRequest (/home/cdswint/services/web/node_modules/@grpc/grpc-js/build/src/client.js:160:34)\n at ServiceClientImpl.crossCopy (/home/cdswint/services/web/node_modules/@grpc/grpc-js/build/src/make-client.js:105:19)\n at /home/cdswint/services/web/server-dist/grpc/vfs-client.js:235:19\n at new Promise (<anonymous>)\n at Object.crossCopy (/home/cdswint/services/web/server-dist/grpc/vfs-client.js:234:12)\n at Object.crossCopy (/home/cdswint/services/web/server-dist/models/vfs.js:280:38)\n at projectForkAsyncWrapper (/home/cdswint/services/web/server-dist/models/projects/projects-create.js:229:19)"]
node:internal/process/promises:288
triggerUncaughtException(err, true /* fromPromise */);
^Error: 4 DEADLINE_EXCEEDED: Deadline exceeded
at callErrorFromStatus (/home/cdswint/services/web/node_modules/@grpc/grpc-js/build/src/call.js:31:19)
at Object.onReceiveStatus (/home/cdswint/services/web/node_modules/@grpc/grpc-js/build/src/client.js:192:76)
at Object.onReceiveStatus (/home/cdswint/services/web/node_modules/@grpc/grpc-js/build/src/client-interceptors.js:360:141)
at Object.onReceiveStatus (/home/cdswint/services/web/node_modules/@grpc/grpc-js/build/src/client-interceptors.js:323:181)
at /home/cdswint/services/web/node_modules/@grpc/grpc-js/build/src/resolving-call.js:94:78
at process.processTicksAndRejections (node:internal/process/task_queues:77:11)
for call at
at ServiceClientImpl.makeUnaryRequest (/home/cdswint/services/web/node_modules/@grpc/grpc-js/build/src/client.js:160:34)
at ServiceClientImpl.crossCopy (/home/cdswint/services/web/node_modules/@grpc/grpc-js/build/src/make-client.js:105:19)
at /home/cdswint/services/web/server-dist/grpc/vfs-client.js:235:19
at new Promise (<anonymous>)
at Object.crossCopy (/home/cdswint/services/web/server-dist/grpc/vfs-client.js:234:12)
at Object.crossCopy (/home/cdswint/services/web/server-dist/models/vfs.js:280:38)
at projectForkAsyncWrapper (/home/cdswint/services/web/server-dist/models/projects/projects-create.js:229:19) {
code: 4,
details: 'Deadline exceeded',
metadata: Metadata { internalRepr: Map(0) {}, options: {} }
}
UPDATE site_config SET grpc_git_clone_timeout_minutes = <new value>;
The ephemeral storage has an upper limit of 10 GB (DSE-31884)
ALTER TABLE
site_config ALTER COLUMN default_ephemeral_storage_limit_mb
property. For
example, to set the limit to 30 GB:
ALTER TABLE site_config ALTER COLUMN default_ephemeral_storage_limit_mb SET DEFAULT 30720;
Enabling Service Accounts (DSE-32943)
Teams in the CML workspace can only run workloads within team projects with the Run as option for service accounts if they have previously manually added service accounts as a collaborator to the team.
Working with files larger than 1 MB in Jupyter causes error (OPSAPS-61524)
While working on files or saving files of size larger than 1 MB, Jupyter Notebook may display an error message such as 413 Request Entity Too Large.
Workaround:
Clean up the notebook cell results often to keep the notebook below 1 MB. Use the
kubectl
CLI to add the following annotation to the ingress corresponding
to the session.
annotations:
nginx.ingress.kubernetes.io/proxy-body-size: "0"
- Get the session ID (the alphanumeric suffix in the URL) from the web UI.
- Get the corresponding namespace:
kubectl get pods -A | grep <session ID>
- List the ingress in the namespace
kubectl get ingress -n <user-namespace> | grep <session ID>
- In the metadata, add the annotation.
kubectl edit ingress <ingress corresponding to the session> -n <user-namespace>
Cannot add the Scala Runtime via UI (DSE-33793)
Users are unable to add the Scala Runtime to their projects explicitly via the UI.
Workaround: User API calls to add the runtime. Alternatively, remove all available runtimes from the list, which makes it possible to create workloads using all runtimes, including Scala.
CML Backup operation fails during validation (DSE-31675)
CML backup operation fails with validation error - "Role validation failed. User must have role 'crn:altus:iam:us-west-1:altus:resourceRole:MLAdmin' for this operation." even though the customer has all the necessary permissions to perform backup. Some customers are facing this issue because of an existing bug in the control plane.
Workaround: Skip the validation when backing up workspace.
Spark executors status reports failed (DSE-32250)
The status of spark executors in the Export Usage List reports failed when completed, regardless of the status of the task.
Terminal does not stop after time-out (DSE-12064)
After a web session times out, the terminal should stop running, but it remaings functional.
CML workspace upgrades disabled with NTP
Upgrades are disabled for CML Workspaces configured with non-transparent proxy (NTP). This issue is anticipated to be fixed in a subsequent hotfix release.
Using dollar character in environment variables in CML
Environment variables with the dollar ($) character are not parsed correctly by CML. For
example, if you set PASSWORD="pass$123"
in
the project environment variables, and then try to read it using the echo command, the
following output will be displayed: pass23
echo 24 | xxd -r -p
or
echo JAo= | base64 -d
$()
or ``
. For example, if you want to set the
environment variable to ABC$123
,
specify:ABC$(echo 24 | xxd -r -p)123
or
ABC`echo 24 | xxd -r -p`123
Models: Some API endpoints not fully supported
In the create_run api
, run_name
is not supported.
Also, search_experiments
only supports pagination.
When a team added as a collaborator, it does not appear in the UI. (DSE-31570)
Run Job as displays even if the job is enabled on a service account. (DSE-31573)
If the job is enabled on a service account, the Run Job as option should not display. Even if me is selected at this point, the job still runs in the service account.
AMP archive upload fails if Project does not contain metadata YAML file
- Download the AMP zip file from GitHub
- Unzip it to a temp directory
- From the command line navigate to the root directory of the zip
- Run this command to create the new zip file:
zip -r amp.zip
.
Make sure you see the .project-metadata/yaml in the root of the zip file.
Cloning from Git using SSH is not supported via HTTP proxy
Workaround: CML Projects support HTTPS for cloning git projects. It is suggested to use this as the workaround.
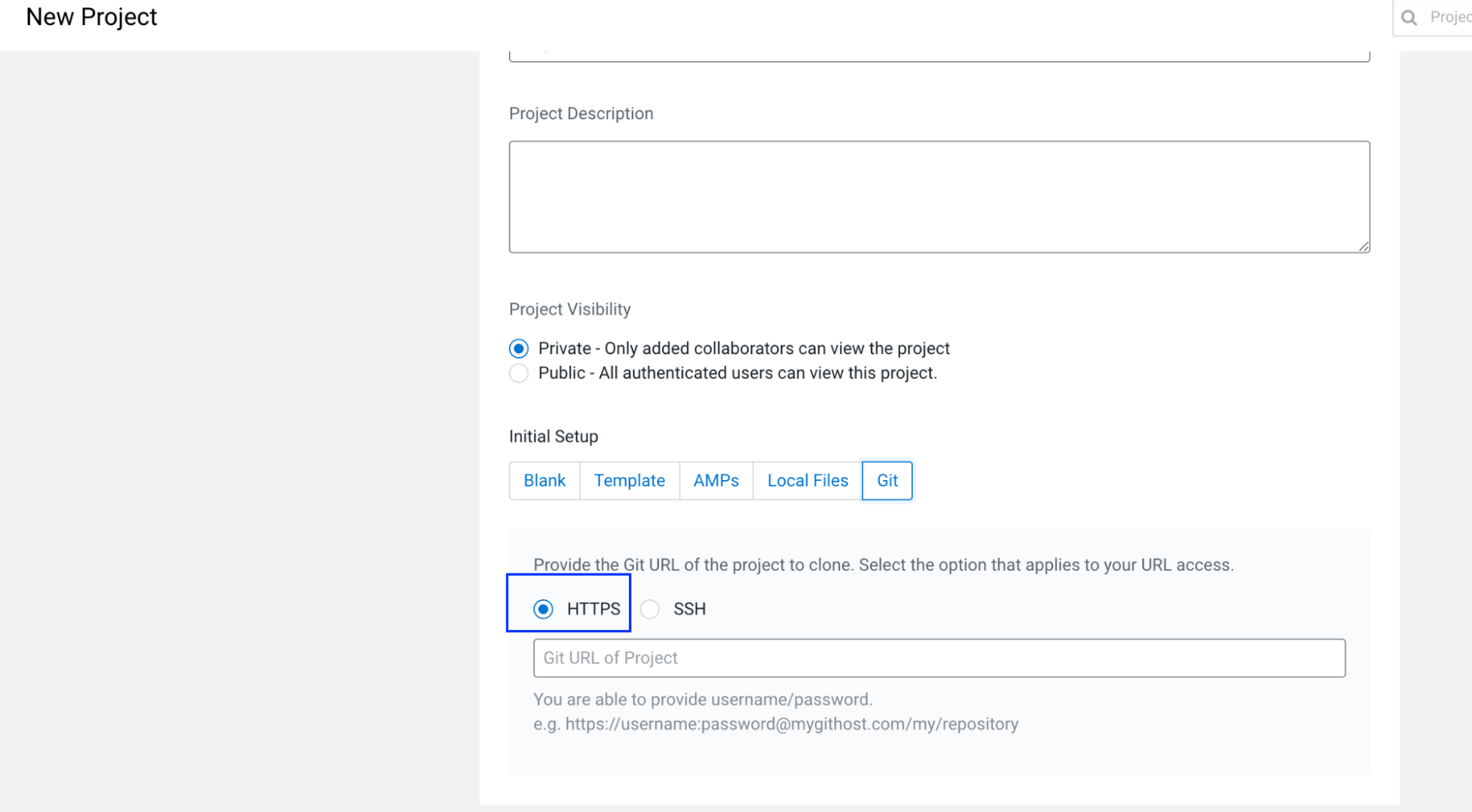
Model deployments requiring outbound access via proxy do not honor HTTP_PROXY, HTTPS_PROXY environment variables
Workaround: Add the HTTP_PROXY, HTTPS_PROXY, http_proxy and https_proxy environment variables to the cdsw-build.sh file of the Project Repository.
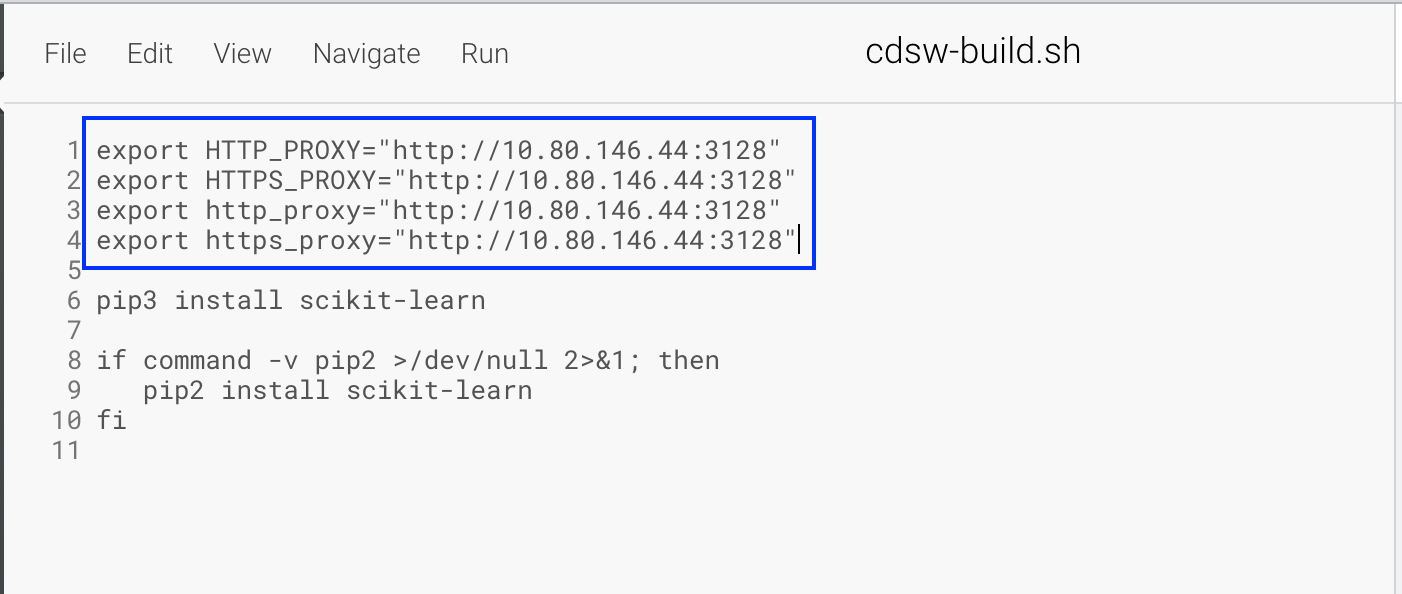
Application does not restart after upgrade or migration
An application may fail to automatically restart after a workspace upgrade or migration. In this case, manually restart the application.
Do not use backtick characters in environment variable names
Avoid using backtick characters ( `
) in environment variable names, as
this will cause sessions to fail with exit code 2.
Model Registry is not supported on R models
Model Registry is not supported on R models.
The mlflow.log_model registered model files might not be available on NFS Server (DSE-27709)
When using mlflow.log_model, registered model files might not be available on the NFS server due to NFS server settings or network connections. This could cause the model to remain in the registering status.
- Re-register the model. It will register as an additional version, but it should correct the problem.
- Add the ARTIFACT_SYNC_PERIOD environment variable to hdfscli-server Kubernetes deployment and set it to an integer value. This will set the model registry retry operation to twice the number of seconds specified by the artifact sync period integer value. If the ARTIFACT_SYNC_PERIOD is set to 30 seconds then model registry will retry for 60 seconds. The default value is 10 and model registry retries for 20 seconds. For example: -name: ARTIFACT_SYNC_PERIOD value: “30”.
Applications appear in failed state after upgrade (DSE-23330)
After upgrading CML from version 1.29.0 on AWS, some applications may be in a Failed state. The workaround is to restart the application.
Cannot use hashtag character in JDBC connection string
The special character #
(hashtag) cannot be used in a password that is
then used in a JDBC connection string. Avoid using this special character, or use
'%23'
instead.
CML workspace installation fails
CML workspace installation with Azure NetApp Files on NFS v4.1 fails. The workaround is to use NFS v3.
Spark executors fail due to insufficient disk space
Generally, the administrator should estimate the shuffle data set size before provisioning the workspace, and then specify the root volume size of the compute node that is appropriate given that estimate. For more specific guidelines, see the following resources.
Runtime Addon fails to load (DSE-16200)
A Spark runtime add-on may fail when upgrading a workspace.
Solution: To resolve this problem, try to reload the add-on. In Reload.
, in the option menu next to the failed add-on, selectCML workspace provisioning times out
When provisioning a CML workspace, the process may time out with an error similar to
Warning FailedMount
or Failed to sync secret cache:timed out
waiting for the condition.
This can happen on AWS or Azure.
Solution: Delete the workspace and retry provisioning.
CML endpoint connectivity from DataHub and Cloudera Data Engineering (DSE-14882)
When CDP services connect to CML services, if the ML workspace is provisioned on a public subnet, traffic is routed out of the VPC first, and then routed back in. On Private Cloud CML, traffic is not routed externally.
NFS performance issues on AWS EFS (DSE-12404)
CML uses NFS as the filesystem for storing application and user data. NFS performance may be much slower than expected in situations where a data scientist writes a very large number (typically in the thousands) of small files. Example tasks include: using git clone to clone a very large source repository (such as TensorFlow), or using pip to install a Python package that includes JavaScript code (such as plotly). Reduced performance is particularly common with CML on AWS (which uses EFS), but it may be seen in other environments.
Disable file upload and download (DSE-12065)
You cannot disable file upload and download when using the Jupyter Notebook.
Remove Workspace operation fails (DSE-8834)
Remove Workspace operation fails if workspace creation is still in progress.
API does not enforce a maximum number of nodes for ML workspaces
Problem: When the API is used to provision new ML workspaces, it does not enforce an upper limit on the autoscale range.
Downscaling ML workspace nodes does not work as expected (MLX-637, MLX-638)
Problem: Downscaling nodes does not work as seamlessly as expected due to a lack of
Bin Packing on the Spark default scheduler, and because dynamic allocation is not currently
enabled. As a result, currently infrastructure pods, Spark driver/executor pods, and session
pods are tagged as non-evictable using the
cluster-autoscaler.kubernetes.io/safe-to-evict: "false"
annotation.
Technical Service Bulletins
- TSB 2023-628: Sensitive user data getting collected in CML/CDSW workspace diagnostic bundles
When using Cloudera Data Science Workbench (CDSW), Cloudera recommends users to store sensitive information, such as passwords or access keys, in environment variables rather than in the code. See Engine Environment Variables in the official Cloudera documentation for details. Cloudera recently learned that all session environment variables in the affected releases of CDSW and CML are logged in web pod logs, which may be included in support diagnostic bundles sent to Cloudera as part of support tickets.
Severity:- Medium
Component affected:- CDSW
- CML workspaces on Public Cloud
- CML workspaces on Private Cloud
Products affected:- Cloudera Machine Learning
Releases affected:- CDSW 1.10.1 and lower
- CML workspaces on Public Cloud 2.0.32-b117 and lower
- CML workspaces on Private Cloud 1.4.0 and lower
Users affected:- CML workspace users who are storing sensitive data like DB passwords or secrets as environment variables in the product.
- CML workspace users who are setting
WORKLOAD_PASSWORD
in CML Public Cloud workspaces from .
Impact:- Session environment variables in CML workspaces (Private Cloud, Public Cloud or OnPrem CDSW) are getting logged and collected when Admin generates diagnostic bundles from . These logs are typically sent to Cloudera as part of support cases.
- On Public Cloud, CML workspace service (web) logs, which are the source of the diagnostic bundles, also get stored on customer S3 or ADLS storage.
Action required: Upgrade CDSW/CML workspace- Upgrade to CDSW 1.10.2 version or higher.
- Public Cloud
- Upgrade Public Cloud CML workspaces to the latest release.
- Delete the CML workspace service logs from S3 or ADLS storage.
- Find the Storage Location for “Logs Storage and Audits” in the Environment service details page. (say <datalake_logs_path>)
- Find the Cluster Name from the CML Workspace details page. (say <cluster_name>)
- Now please delete all the files under <datalake_logs_path>/<cluster_name> folder that are generated before CML workspace is upgraded to the latest with this fix.
- Private Cloud
- Upgrade Private Cloud CML workspace to 1.4.1 or higher
Addressed in release/refresh/patch:- CDSW 1.10.2
- Public Cloud 2.0.32-b123
- Private Cloud 1.4.1
- Knowledge article
- For the latest update on this issue see the corresponding Knowledge article: TSB-2023-628: Sensitive user data getting collected in CML/CDSW workspace diagnostic bundles
- TSB 2022-588: Kubeconfig and new version of aws-iam-authenticator
Regenerate Kubeconfig and in conjunction use a newer version of aws-iam-authenticator on AWS. Kubeconfig in Cloudera Data Platform (CDP) Public Cloud Data Services needs to be regenerated because the Kubeconfig generated before June 15, 2022 uses an old APIVersion (client.authentication.k8s.io/v1alpha1) which is no longer supported. This causes compatibility issues with aws-iam-authenticator starting from v0.5.7. To be able to use the new aws-iam-authenticator, the Kubeconfig needs to be regenerated.
Severity:- High
Component affected:- Cloudera Machine Learning (CML) Data Service
- Cloudera Data Engineering (CDE) Data Service
- Cloudera Data Flow (CDF) Data Service
Products affected:- CDP Public Cloud
Releases affected:- All existing and upcoming CDP Public Cloud releases using the affected components, listed above.
Users affected:- Users of CML, CDE, and CDF using Kubeconfig generated before June 15, 2022 and a version of aws-iam-authenticator prior to v0.5.7.
Impact:- Customers using a Kubeconfig generated before June 15, 2022 and an aws-iam-authenticator version prior to v0.5.7 may see Kubernetes clients not able to access the cluster successfully.
Action required:
From June 15, 2022 onwards, existing customers on AWS using a previously generated Kubeconfig will have to:- Regenerate and use the new Kubeconfig, and
- Use a new version of aws-iam-authenticator starting with v0.5.7.
The newly generated Kubeconfig changes the APIVerson of the user’s section as:- Old:
- ApiVersion: "client.authentication.k8s.io/v1alpha1"
- New:
- ApiVersion: "client.authentication.k8s.io/v1beta1"
- Knowledge article
- For the latest update on this issue see the corresponding Knowledge article: TSB-2022-588: Kubeconfig and new version of aws-iam-authenticator